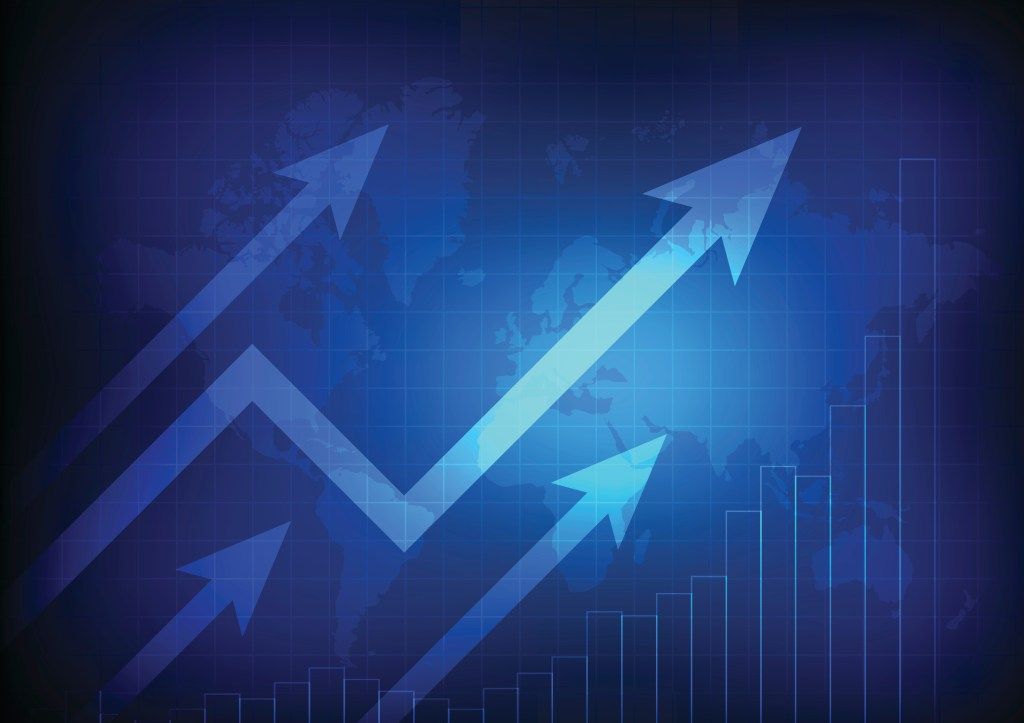
Inference-Time Search: A New AI Scaling Law or Just a Workaround?
The AI world is buzzing about a potential new "scaling law" called "inference-time search." But what is it, and is it really a game-changer? Let's dive in.
What is Inference-Time Search?
AI scaling laws describe how AI model performance improves with increased dataset size and computing power. While pre-training was once the dominant approach, post-training scaling and test-time scaling have emerged. Now, researchers at Google and UC Berkeley propose "inference-time search" as a potential fourth law.
Inference-time search involves generating multiple possible answers to a query and then selecting the "best" one. Researchers claim this method can significantly boost the performance of models like Gemini 1.5 Pro, even surpassing OpenAI's o1-preview on certain benchmarks.
Eric Zhao, a Google doctorate fellow and co-author of the paper, explained on X that "by just randomly sampling 200 responses and self-verifying, Gemini 1.5 — an ancient early 2024 model — beats o1-preview and approaches o1." He also noted that self-verification becomes easier at scale.
Skepticism from Experts
Despite the initial excitement, some experts remain skeptical about the widespread applicability of inference-time search.
Matthew Guzdial, an AI researcher at the University of Alberta, points out that this approach works best when a good "evaluation function" is available, meaning the best answer can be easily identified. However, many real-world queries don't have such clear-cut solutions.
"[I]f we can't write code to define what we want, we can't use [inference-time] search," Guzdial explains. "For something like general language interaction, we can't do this […] It's generally not a great approach to actually solving most problems."
Mike Cook, a research fellow at King's College London, echoes this sentiment, emphasizing that inference-time search doesn't necessarily improve the model's reasoning process. Instead, it's a workaround for the limitations of AI, which can sometimes make confident but incorrect predictions.
"[Inference-time search] doesn't 'elevate the reasoning process' of the model," Cook said. "[I]t's just a way of us working around the limitations of a technology prone to making very confidently supported mistakes."
The Search Continues
The limitations of inference-time search may disappoint those seeking more efficient ways to scale model "reasoning." As the researchers themselves acknowledge, current reasoning models can be incredibly computationally expensive. Therefore, the quest for new and effective scaling techniques remains a priority in the AI field.
Source: TechCrunch